The semiconductor industry in China faces mounting challenges as it navigates international pressures and evolving market demands. Recent developments underscore a tightening grip on the global semiconductor landscape, with a significant statement released on December 3 by four major associations, including the China Semiconductor Industry Association. This statement urges local Chinese enterprises to tread cautiously when procuring American-made chips, signaling a shift in the procurement landscape.
In an era where artificial intelligence (AI) and the digital economy are proliferating at an unprecedented pace, the demand for AI chips and the computational power they provide has surged. These chips have become essential in driving global digital and smart transformation, acting as a key to unlock new economic potential. According to estimates from the China Academy of Information and Communications Technology, every one yuan invested in computing power can stimulate a GDP growth of three to four yuan, showcasing the powerful economic engine that is computational infrastructure.
Advertisement
Meanwhile, despite facing numerous setbacks, China's AI sector is leveraging this momentum to hasten its path toward self-reliance. The nation has witnessed a meteoric rise in its computational capabilities, currently boasting a total computational scale of 246 exaFLOPS, which places it among the leaders in the global arena. Projections suggest that by 2025, the core industry of computational power will balloon to a staggering 4.4 trillion yuan, with the related industrial ecosystem anticipated to exceed 24 trillion yuan.
As this growth unfolds, the scenario in China is witnessing substantial changes; however, the burgeoning domestic computational capabilities are now encountering a new set of challenges. Issues such as supply-demand mismatches, underutilized computational resources, and low utilization rates are beginning to surface as significant hurdles in the operational landscape.
Consequently, the demand for "computational operations" is on the rise, with market analysts echoing this sentiment. Data from IDC indicates that China's intelligent computational service market reached nearly 20 billion yuan in 2023, and is set to maintain an impressive compound annual growth rate of 18.9% over the next five years, potentially reaching the 307.5 billion yuan mark by 2027.
The increasing uncertainty in the global market underscores the need for the commercialization of domestic chips, revealing a critical opportunity in the untapped "computational operations" market, which is being explored by several forward-thinking enterprises. These companies are strategically working towards facilitating high-quality, self-sustained growth in China's core chip industry. The pressing questions remain: how can idle computational resources be effectively activated? How can computational strength further energize the AI market?
In light of these complexities, the "First New Voice" team has interviewed industry experts, including Sun Rongfeng, head of the strategic development department at Wuxi Data Group, Wang Sheng from Inno Angel Fund, and Guo Liang, chief engineer at the Cloud Computing and Big Data Institute of the China Academy of Information and Communications Technology, among others, to gain insights into the new opportunities and solutions in the realm of computational power.
01: Is Computational Power Languishing?
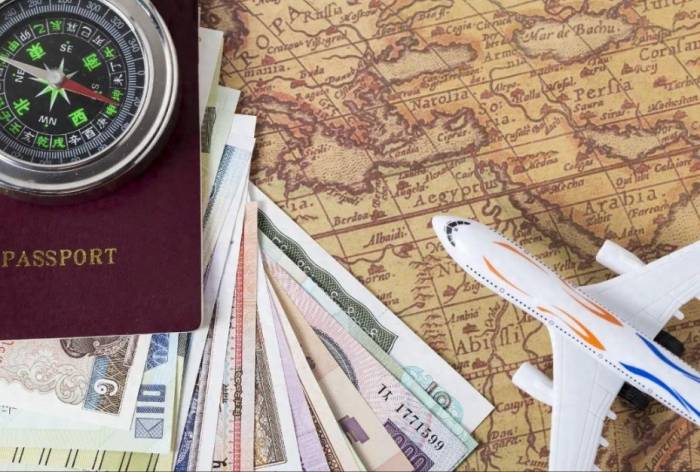
Amid the fervor of computational power infrastructure development, an unsettling shift towards underutilization has emerged. The explosion of large-scale models has indeed triggered a surge in the need for computation, prompting local governments, telecom operators, and tech giants to fast-track the construction of intelligent computational centers to capitalize on this burgeoning demand. Data indicates that the number of intelligent computational centers in China surged to over 250 by the first half of this year, compared to just around 30 centers a year prior.
The influx of new computational capacity has raised fresh questions about the market's adaptability. What changes have unfolded as the computational wave crashes into the market? Observations from both supply and demand dynamics of intelligent computational centers and major model-driven firms can provide clarity.
Initially, a warning signal regarding "idle computational power" has been sounded within many newly built intelligent computational centers. Despite the rapid construction, many centers are finding that this newly added computational power is not being optimally utilized for practical applications or to assist local industrial upgrades. “Currently, 90% of the intelligent computational centers in the country have a capacity below 1000P, which has limited efficacy in training large models, with future efficiency remaining dubious,” cautions Guo Liang from the China Academy of Information and Communications Technology.
From the latter half of 2024, the issue of vacant server racks within these centers has become increasingly apparent. A notable computational operator in Beijing noted a growing sense of urgency around the need for market-driven solutions to address this challenge after engaging with numerous local governments and enterprises launching intelligent computational centers.
One manager at a large intelligent computational center shared: “Starting in 2024, there has been a clear decline in the number of enterprises looking to purchase or lease computational hardware; even merely competing on price has proven ineffective in absorbing the existing capacity.”
At this moment, the majority of these centers rely heavily on large model training customers—known for their substantial computational power consumption—but the challenges are evident: high-profile clients are dwindling, and many centers cannot offer sufficiently attractive pricing or strategies to appeal to medium and small-sized enterprises, thus leading to a standstill in effective capacity utilization.
A consensus is emerging that B-to-B consumers are inclined to select familiar partners, with successful transactions often limited to established relationships or companies with robust overall capabilities, as highlighted by Zhang Yazhou of Shanghai Runliuchu Technology Co. This scarcity of high-value clientele poses additional challenges for newly established intelligent computational centers in their quest for customers.
Furthermore, the demand for large model training has noticeably cooled, while the growth in inference demand is a gradual process, indicating a broader cooling phase in the overall computational procurement market.
Having experienced two years of rapid growth, the large model sector's fervor seems to be cooling. Internationally, companies like OpenAI and Anthropic have repeatedly delayed the rollout of their latest models, while Chinese firms in the same sector are increasingly adopting a minimalist approach.
While the release of GPT-5 continues to be postponed, the lack of technical leadership in the market limits the vibrancy of large model development and training. Coupled with high training costs and ongoing risks associated with open source technology, the industry is turning its gaze toward the next generation of large models, hoping for a novel framework to reinvigorate market activity.
Recent statistics reveal that by October 2024, 188 large models had registered through generative AI; however, over one-third of these models have made no public progress post-registration, and only about 10% are actively undergoing accelerated training.
On another front, there's a growing recognition within the industry that larger models do not necessarily equate to superior performance, as outlined by former IDC analyst Jin Lei. “Some large model firms are shifting their focus to sector applications after their foundational models reach 10 billion parameters, rather than relentlessly pursuing rankings based on parameter count,” he explained.
Moreover, large model enterprises are refocusing on their core strengths after extensive commercial explorations in various fields. For instance, in September of this year, the company Dark Side of the Moon ceased work on two overseas projects, Ohai and Noise, to focus solely on the development of Kimi. Wang Xiaochuan, founder of Baichuan Intelligence, emphasized a commitment to AI in healthcare. As Yao Yi, founder and CEO of First New Voice put it, “These moves towards sector specializations and scaled-back initiatives are fundamentally about controlling costs to achieve true commercial viability. The destructive cash-burning strategy of the previous competition in the large model sector has reached its conclusion, with a focus on efficiency emerging as the more prudent approach.”
Meanwhile, investors are adjusting to a more rational perspective on funding some market-leading models, indicating that the computational market may face short-term pressure until a significant inference demand materializes.
The current trajectory also points to a significant challenge—the shortage of safe and reliable high-quality datasets. As Li Zhe, head of AI technology at Ant Group, noted, “Future AI applications will require vast quantities of rare and hard-to-obtain long-tail data, such as extreme weather and road conditions for autonomous driving, or complex scene data necessary for embodied intelligence training.”
In recent years, as large model technology has developed, machine learning is shifting its focus from being model-centric to being data-centric. High-quality data can significantly enhance model accuracy and stability, yet as the present state illustrates, a lack of sufficient data is now a critical bottleneck hampering model evolution. According to predictions from Gartner, by 2024, 60% of AI data will consist of synthetic data, while Epoch AI Research projects that existing high-quality language data for AI model training will be exhausted by 2026.
02: The Dilemma of "Buying" and "Selling": Market Trapped in Low Efficiency
The situation is awkward in the computational market, where the utilization rates of intelligent computational centers are languishing while numerous small enterprises struggle to afford the exorbitant costs associated with computational power.
IDC's latest surveys reveal that utilization rates for computational centers geared towards enterprise users regularly hover around a low 10%-15%. By comparison, to generate meaningful economic returns, an ideal usage rate for such centers would be at least 80%. As a surplus of computational resources fall prey to a “sleeping crisis,” those in need of computational resources find it difficult to secure suitable options in the marketplace. “Although computational prices have seen some declines this year, for many small and medium enterprises, it is still prohibitively expensive,” Yang Zhen, head of strategic and market development at BD Intelligence, pointed out, highlighting the invisible barriers that inhibit transactions between supply and demand parties.
Jin Lei further elucidated, “The key reasons for idle computational power stem from one party 'not being able to buy' and the other 'not able to sell'. Several underlying factors contribute to this predicament: first, restrictions on the purchase of imported chips, coupled with a performance gap in domestic chips that dampens market enthusiasm; and second, numerous intelligent computational centers prefer a single GPU cluster model, which is often inadequate to meet diverse industrial needs at the local level. Lastly, traditional leasing and sales models restrict these centers' ability to cater to a broader client base.”
For instance, the challenges in importing chips alongside mediocre performance of domestic alternatives result in a lack of compelling usage data, thereby complicating procurement choices, which is undeniably a key reason why computational power isn't being utilized efficiently.
The prevalent supply constraints surrounding international chips have opened gaps that domestic options must fill. Furthermore, bolstered by supportive policy initiatives, domestic computational capabilities are progressively securing a larger share of the market. Nevertheless, as observed by Zhang Yazhou, participation from a wide spectrum of domestic computational power players, including computer equipment manufacturers and ICT communication companies, remains largely unfounded with only a few achieving tangible outcomes. Renowned Academy of Engineering member Liu Yunjie pointed out at the 2024 China Computational Power Conference, “Domestic computational power has reached a certain scale, but utilization rates are far from ideal.”
“At present, the challenges associated with the implementation of domestic GPU and AI chip companies are exceedingly high. For a domestic chip to find its place within an intelligent computational center, it must help secure a customer company willing to cover the costs of the chip and equipment,” elaborated Wu Yue from BD Intelligence's industrial ecosystem team. This highlights a tightly interwoven chain linking chip manufacturers, computational centers, model developers, and end customers.
Moreover, the current limitations of the three main supply models in computational power further exacerbate the issues surrounding supply and demand mismatches.
Currently, the predominant market models for supplying computational power consist of three types: the first involves government-run or state-owned enterprises building computational centers for investment attraction or industry guidance; the second entails large model companies owning their computational centers primarily for their needs, providing excess capacity to other market players through cloud rental services; and the third includes service providers constructing public computational centers designed to aggregate idle computational resources and align them with customer needs.
The common thread among these models is that most rely on an "exclusive" leasing or sales framework, meaning that even during periods of non-usage, fees continue to accrue irrespective of actual consumption. This exclusive model tends to foster resource underutilization, redundancy, and waste. Yao Yi believes this method is practical for large parameter models training but unsuitable for public computational service provision.
“There is significant demand for computational power; however, the existing supply types are insufficient to meet user expectations in terms of adaptation and cost-effectiveness,” summarized IDC's Chinese analyst Du Yunlong. As AI companies grapple with soaring computational costs and small developers and startups encounter particularly acute pressure in leasing computational resources, raising efficiency and making computational power more easily accessible for smaller companies and individual developers is vital for industry advancement.
03: Bridging the Islands of "Computational Power, Algorithms, and Data" is Critical
While foundational computational infrastructure continues to improve, these resources resemble isolated "chimneys," constructed independently and lacking the necessary connectivity and bridges to enhance utility across various supply chains, leading to excessive resource waste.
Recent discussions at the Baidu Intelligent Cloud technology forum highlighted a critical issue: “the effective utilization rate of computational power in large model training remains under 50%.” This discourse rekindled the focus on how to increase the effective use of computational power within the industry at large.
The "chimney problem" affecting the computational market is driven by multifaceted factors straddling AI industry dynamics and national socioeconomic contexts. As Yang stressed, resolving the issue of locked-away computational resources fundamentally necessitates industry-level solutions. The critical trio of AI requirements—computational power, algorithms, and data—are interconnected; thus, innovative coordination across all three channels is essential to enhance the efficiency of resource allocation and execution in the industry.
In terms of computational power, solutions have been proposed to address the performance shortfalls of domestic chips and the limitations associated with single-GPU clusters through the establishment of “heterogeneous hybrid clusters.”
Currently, stark performance disparities exist between domestic and foreign chips; single-brand chip clusters suffer from fixed weaknesses. “By integrating weaker and robust chips into a heterogeneous cluster and employing algorithmic adaptations to approximate the performance of high-end chips, we can exceed the restrictions of single clusters and achieve enhanced collaborations,” Jin Lei notes.
However, creating effective multi-card clusters requires overcoming challenges related to technical complexities, resource allocation, and ecological support. Despite many companies claiming to offer multi-cluster management capabilities, the reality is that very few can deliver results, as Yang pointed out: “genuine collaborative efforts across multiple clusters are rare, and much of heterogeneous computation is limited to connectivity between two clusters.” BD Intelligence is responding to this with its "Progress·AI Heterogeneous Computing Platform," which aims to advance scalable multi-cluster collaborations and has already set up three domestic heterogeneous clusters, which upon full-scale operation, will yield up to 2000 PFLOPS of intelligent computational supply.
“Minimizing computational latencies and allowing diverse computational clusters to function in a highly adaptive and cooperative manner is poised to be a key developmental trend for the next stage,” Wu Yue remarks. This involves meticulous work—ensuring that algorithm libraries and communication frameworks are fully established is imperative to ensure that chips can effectively support disparate foundational large models.
Simultaneously, in contrast to the constraints of traditional leasing and sales models, adopting a token-based pricing strategy could significantly reduce the costs associated with computational power usage. “The operational aim of computational centers should be to offer users fundamental computational support comparable to utilities like water and electricity, charging fees only when computational resources or model services are actually utilized, thus enabling users to plug and play,” Wu Yue explains. Using token-based pricing models primarily serves to assist small enterprises in overcoming prevalent computational application challenges, especially when training vertical models for traditional clients such as hospitals, where costs can even drop to one-tenth of previous rates.
In the realm of algorithms, executing professional algorithms atop robust heterogeneously configured clusters allows for optimized scheduling, ensuring the stability of cross-cluster training and addressing the disconnections between computational strength and models.
Consequently, various models typically come equipped with their respective ecosystems, adaptation chips, and development frameworks, resulting in some level of closure. Consequently, companies face significant hurdles due to the notable discrepancies in their ecological systems, rendering model migration to alternate computational chips arduous. The process involves both performance variances and heightened costs, potentially resulting in compatibility gaps during model migrations, along with considerable trial-and-error investments—these factors often deter multiple clients from adopting domestic computational solutions.
The cornerstone product of BD Intelligence, the "Baota·Model Adaptation Platform," constructs an adaptation layer akin to an operating system, providing a unified interface for different hardware to ensure compatibility with mainstream products such as those from NVIDIA. “By adapting downwards to various chips and upwards to differing frameworks, this ubiquitous solution effectively dismantles barriers across chips, models, and development frameworks. In this platform, any chip or model can be deployed and developed without reserve, allowing clients to bypass concerns regarding underlying hardware intricacies while consistently adhering to a standard interface—ultimately resolving the prevalent low utilization of computational power in the market,” Yang describes.
This mixed-batch strategy promotes hybrid utilization across various computational resources and will markedly enhance the efficiency of both model training and inference tasks. “In training scenarios, hybrid technology can address the migration and coordination quandaries that arise between diverse computations. Concurrently, in inference tasks, the same technology can be harnessed to intelligently allocate high-performance chips for initial tokens while tapering off to lower-performance chips for subsequent processing. This ensures that computational consumption is minimized while maintaining a quick inference speed,” Jin Lei adds.
According to calculations, by implementing the "Progress" and "Baota" platforms onto existing intelligent computational centers, operational efficiency could double, while the efficiency for pure inference requests could surge by over 300%. “Moreover, by leveraging software optimizations, we can enhance the performance of domestic chips while prolonging their lifecycle. Should models lack support for user frameworks, we can also connect with open-source model libraries to facilitate a quick development process for users with little to no coding,” Yang highlighted.
Turning to data, challenges regarding data collection, effective utilization, and credible datasets have emerged as substantial roadblocks preventing large model advancements, making it imperative to resolve these data dilemmas as a prerequisite for improving training quality and thereby increasing computational efficiency.
Recently, Yu Xiaohui, President of the China Academy of Information and Communications Technology, emphasized the necessity of building a “data space” to enhance the role of data factors. Within the current data crisis, distribution challenges are paramount. Reports indicate that approximately 70% of high-quality data in China is controlled by governmental and enterprise entities. However, leveraging this data faces several hurdles: concerns surrounding data security and compliance with national standards hinder access, while the absence of an effective mechanism and platform to ensure data integrity and monetary value further limits market transactions.
When these distribution dilemmas propagate to small model manufacturers and developer teams, data seekers find themselves grappling with difficulties in procuring suitable training environments, hindering technological innovations from reaching practical applications. Thus, creating a digital infrastructure that guarantees safe, lawful, and credible data exchanges—a “trustworthy data space”—has become an urgent necessity.
“Currently in Wuxi, there are not many platforms providing data for AI,” stated Sun Rongfeng, head of strategy development at Wuxi Data Group, discussing the status of data transactions in the area. As a manufacturing powerhouse, Wuxi has seen limited participation in providing data resources for AI models due to the complexities involved in data authentication, governance, cleaning, and calibration processes.
To explore local data potentials and empower industrial upgrades, Wuxi Big Data Group has taken on the role of “developing” and “operating” public data resources across various sectors. They are actively building a data trading ecosystem to facilitate the commercialization and flow of data. This includes collaborating with data trading platforms in larger cities like Shanghai and Shenzhen to process and develop public data products to integrate local data resources into broader provincial and national markets,” Sun shared.
Historically, data trading platforms have operated through direct supply APIs or offline approval processes with non-managed raw data, which has left significant compliance and safety holes. Such methods have been cumbersome, while the true value of data has largely been underexploited. BD Intelligence's "Honghu·Trustworthy Data Space" directly addresses these transaction barriers, ensuring data safety and securing stakeholder interests through multi-tiered solutions and sustainable operational models that form a complete commercial ecosystem.
“For example, in embodied intelligent applications, the backing of a trustworthy data space enables data to enter training environments, infuse models, and even become embedded within integrated devices. As data scenarios proliferate and new data constantly flows in, a trustworthy data space can ensure steady earnings for data providers while enhancing model quality, accuracy, and the diversity of application scenarios for data users,” Yang assessed. This model holds promise for further maturation as the data trading market expands.
04: The Market Calls for an “Industrial Ecosystem” and the Need for “String Pullers” in the Industry
“The computational power, algorithms, and data within the AI market are like scattered pearls, and the industry needs a ‘string puller’ role to connect these beads and tighten the links between the existing components of the supply chain,” Yang Zhen believes. Intelligent computational centers, while being capital-intensive ventures, are only able to retain minimal profit margins, primarily due to their separation from final business scenarios and the low level of influence they hold within the industry chain.
If intelligent computational centers aspire to break through and pursue deeper levels of development, Wu Yue suggests two pathways: one is to build an ecosystem to serve a wider array of small and medium enterprise clients through all-encompassing capabilities, and the other entails establishing substantial scales of over ten thousand units to cater to a select few clients.
The essential market demand pertains to comprehensive solutions rather than individual products or year-long subscriptions; thus, the operation of intelligent computational centers is fundamentally about managing the AI industry supply chain. To capture a substantial market share and secure industry profits, these centers must dive deeper into the computational sector, transitioning from pure computational services to delivering tailored, value-added services and individualized solutions that effectively align with business contexts.
“For most small to medium intelligent computational centers, integrating into an industrial ecosystem may well be the only viable solution,” Jin Lei stated.
Thus, creating a robust and sustainable industrial ecosystem becomes paramount for fostering the continuous and healthy development of the computational power market. Looking ahead, what kind of computational ecosystem should be constructed, and how can it spur the ongoing growth of the AI industry?
Yang Zhen argues, it is imperative for computational operators to embody the role of the “string puller” by aggregating idle computational resources and tailoring solutions to meet clients' specific needs.
However, constructing a platform that effectively links all parties in the computational power sector and the broader AI market can be extraordinarily challenging. Operators must closely examine barriers across the four dimensions of computational power, algorithms, data, and scenarios while leveraging a full-stack AI layout to surmount various obstacles. By doing so, they can assist intelligent computational centers in integrating local computational demands with regional industrial structures, ultimately creating unique AI operational centers that drive effective absorption of idle computational resources and amplify the industry-empowering potential of AI infrastructure.
At this stage, intelligent computational centers nationwide are becoming increasingly aware of the significance of industrial ecosystems and are initiating their pursuits in this domain. For instance, BD Intelligence's “Xinghuo·Intelligent Calculation” boasts an all-encompassing capability spanning from chip development and algorithm implementation to trustworthy data space establishment. Additionally, the primary project under Xinghuo·Intelligent Calculation—the Beijing Digital Economy Computational Center—has shattered traditional frameworks by incorporating functionalities such as computational exhibitions, innovative labs, and incubation platforms into the intelligent computational center. This multifaceted approach enhances the connection between industry elements while fostering technological innovations and yielding a healthy circular growth within the ecosystem.
“Xinghuo·Intelligent Calculation is not merely a physical structure; it represents a fusion of ‘intelligent computational centers’ and ‘industrial ecosystems’. Through robust computational support systems, generic algorithms, high-quality data tools, and an open mindset towards ecological formations, it equips intelligent computational centers to evolve from mere utilitarian layers to holistic ecological models that can adapt to the needs of customer bases,” Yang elaborated. So far, BD Intelligence has partnered with approximately 1000 ecosystem allies, with plans to establish and implement three to four Xinghuo·Intelligent Calculation centers across various localities, aimed at broadening the potential scale of impacts and engagements.
In conclusion, Sun Rongfeng found this ecosystem-oriented model favorable: “Fostering an industrial ecosystem is an effective approach to addressing the ongoing supply-demand conflicts evident in numerous cities, particularly for small and medium cities like Wuxi, which can benefit greatly from this model by integrating diverse computational provisions to tackle fragmentation issues arising from their limited computational capabilities.”
Regarding concrete industrial models, Yang Zhen proposed two directions: first, government and state-owned enterprises can drive effective digital transformations in local industries by constructing and operating public intelligent computational centers; second, small to medium-sized enterprises can achieve maximal benefits through active participation in the computational power industrial ecosystem, facilitating the establishment of a healthy and sustainable AI development framework.
On one hand, current government measures, such as issuance of computational power coupons and investment promotion policies, are not fundamentally addressing the absorption challenges faced by local centers. The promising solutions lie in addressing root causes. Many intelligent computational center projects are primarily driven by local governments and investment platforms, thus opening the field for AI ecosystems should commence with governmental actions to unveil data and opportunities within existing business scenarios. By harnessing state enterprises to harness vertical models, initial growth can be generated while more granular operational oversight is employed to elevate computational utilization and lower operating costs. Subsequently, integration of supply and demand, while accounting for regional industrial characteristics, can lead to more effective AI transformation and characteristic development in regional AI sectors.
Proactive cities are already keenly recognizing these opportunities and are embarking on their own explorative paths. Sun Rongfeng articulated the current data landscape in Wuxi, revealing that much of the data housed on the local trading platform can significantly contribute to training AI models. In this light, Wuxi Big Data Group has embarked on projects to develop local government models while vigorously exploring vertical models pertinent to AI.
On the other hand, many vertical domains within AI remain marred by breakages and bottlenecks within their supply chains, leading numerous high-potential AI startups to fade into oblivion merely before establishing viable business models.
In the case of embodied intelligence, a plethora of small-to-medium enterprises and individual developers are filling niche markets that larger corporations typically avoid. However, they encounter dual challenges stemming from high computational costs and expensive data acquisition throughout their development processes, which further complicates the path for the entire industry chain's deployment.
Similarly, domestic computational chips have experienced swift growth in recent years; however, the absence of comprehensive application demonstrations and effective evaluation frameworks has led to unclear perceptions regarding operational capabilities among computational adopters.
In light of these dilemmas, BD Intelligence strongly advocates for constructing a robust computational and AI industry ecosystem, which can bridge prevailing gaps. Such construction would facilitate access to affordable, adaptable computational power while enabling myriad AI applications for small enterprises and individual developers, ultimately accelerating the commercialization of products and maximizing operational effectiveness within the sector.
In a bid to ameliorate the existing dearth of comprehensive application demonstrations and effective evaluation mechanisms surrounding domestic computational power platforms, BD Intelligence has unveiled the “first domestic computational power PoC platform” which is now operational. This pioneering initiative leverages the Progress·AI Heterogeneous Computing Platform to facilitate scaled testing in production environments. By innovatively instituting an "evaluation-driven engagement" model, the platform also accommodates industry vertical scenarios and validation services while promoting adaptation and integration of various computational resources across finance, governance, industry, healthcare, and embodied intelligence application sectors—eventually facilitating seamless, bidirectional linking of foundational computational resources to business scenarios and propelling domestic computing capabilities from merely “available” to “user-friendly.”
“The AI industry necessitates collective efforts to accelerate growth. It’s crucial to cooperate, especially as artificial intelligence reshapes the technological landscape in the industry, collaboration will naturally pave the way for breakthroughs,” Yang Zhen emphasizes. When artificial intelligence solidifies its status as a globally strategic field and as the evolution of large models transitions into the “post-training” phase, the Chinese AI sector must unite in discourse and cooperation to witness and manifest the advent of a new intelligent epoch.
Post Comment